Bachelor/Master Theses, Semester Projects, and DAS DS Capstone Projects
If you are interested in one of the following topics, please send an email to Prof. Bölcskei and include your complete transcripts. Please note that we can not respond to requests that are sent directly to PhD students in the group or do not contain your transcripts.
These projects serve to illustrate the general nature of projects we offer. You are most welcome to inquire directly with Prof. Bölcskei about tailored research projects. Likewise, please contact Prof. Bölcskei in case you are interested in a bachelor thesis project.
Also, we have a list of ongoing and finished theses on our website.
List of Bachelor Projects (BA)
- Why do neural networks generalize?
- Reconstruction and classification of eclectrocardiograms with transformers
List of Semester Projects (SP)
- Why do neural networks generalize?
- Approximation rates of transformer networks
- Acoustic sensing and trajectory estimation of objects flying at supersonic speed (with industry)
- Separability of structured data
- Complexity of ꞵ-expansions
- Continuous-time recurrent neural networks in Banach spaces
- General signal denoising
- Reconstruction and classification of eclectrocardiograms with transformers
List of Master Projects (MA)
- Learning in indefinite spaces
- Automatic synopsis generation from amendment proposals for German law
- Why do neural networks generalize?
- Approximation rates of transformer networks
- Complexity of ꞵ-expansions
- Learning cellular automaton transition rules with transformers
- Finite-precision neural networks
- General signal denoising
- The "logic" behind transformers
- Reconstruction and classification of eclectrocardiograms with transformers
Learning in indefinite spaces (MA)
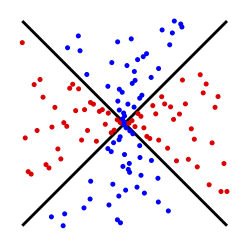
However, in many applications the kernel function fails to be positive semidefinite [2] which, in turn, leads to so-called (indefinite) Krein spaces [3]. The goal of this project is to develop a theory of learning for reproducing kernel Krein spaces.
Type of project: 100% theory
Prerequisites: Strong mathematical background, measure theory, functional analysis
Supervisor: Erwin Riegler
Professor:
Helmut Bölcskei
References:
[1] F. Cucker and D. X. Zhou, "Learning theory," ser. Cambridge Monographs on Applied and Computational Mathematics, Cambridge University Press, 2007.
[2] R. Luss and A. d’Aspremont, "Support vector machine classification with indefinite kernels," Mathematical Programming Computation, vol. 1, no. 2-3, pp. 97–118, Oct. 2009.
[3] A. Gheondea, "Reproducing kernel Krein spaces," Chapter 14 in D. Alpay, Operator Theory, Springer, 2015.
Automatic synopsis generation from amendment proposals for German law (MA)
Recently significant advances in machine translation and question answering were made using transformer networks that are pretrained on large unsupervised data sets [4, 5, 6]. Machine learning solutions for the specific task at hand have, however, not been studied previously. Significant new contributions will hence be required. In particular, the semi-structured nature of amendments might make it necessary to incorporate a copy mechanism [7, 8, 9]. In this project, you will have the opportunity to, first, make novel contributions to the field of natural language processing and, second, to develop a working algorithm that can be deployed online and used by the general public.
Type of project: 70% implementation/programming, 30% model development
Prerequisites: Experience with deep learning for natural language processing (NLP), knowledge of German
Supervisor: Clemens Hutter, Joseph Rumstadt
Professor:
Helmut Bölcskei
References:
[1]
"Gesetz zur Modernisierung des notariellen Berufsrechts und zur Änderung weiterer Vorschriften."
[Link to Document]
[2] F. Herbert, "Verfassungsblog: On matters constitutional," 2021, doi: 10.17176/20210305-033813-0. [Link to Document]
[3] "Synopse: Gesetz zur Modernisierung des notariellen Berufsrechts und zur Änderung weiterer Vorschriften." [Link to Document]
[4] A. Vaswani, N. Shazeer, N. Parmar, J. Uszkoreit, L. Jones, A. Gomez, L. Kaiser, and I. Polosukhin, "Attention is all you need," Advances in Neural Information Processing Systems, pp. 5999–6009, 2017. [Link to Document]
[5] A. Radford, T. Narasimhan, T. Salimans, and I. Sutskever, "Improving language understanding by generative pre-training," Preprint, pp. 1–12, 2018. [Link to Document]
[6] J. Devlin, M. Chang, K. Lee, and K. Toutanova, "BERT: Pre-training of deep bidirectional transformers for language understanding," NAACL HLT 2019 - 2019 Conference of the North American Chapter of the Association for Computational Linguistics: Human Language Technologies - Proceedings of the Conference, vol. 1, pp. 4171–4186, 2019. [Link to Document]
[7] J. Gu, Z. Lu, H. Li, and V. Li, "Incorporating copying mechanism in sequence-to-sequence learning," 54th Annual Meeting of the Association for Computational Linguistics, ACL 2016 - Long Papers, vol. 3, pp. 1631–1640, 2016, doi: 10.18653/v1/p16-1154. [Link to Document]
[8] A. See, P. Liu, and C. Manning, "Get to the point: Summarization with pointer-generator networks," ACL 2017 - 55th Annual Meeting of the Association for Computational Linguistics, Proceedings of the Conference (Long Papers), vol. 1, pp. 1073–1083, 2017, doi: 10.18653/v1/P17-1099. [Link to Document]
[9] B. McCann, N. Keskar, C. Xiong, and R. Socher, "The natural language decathlon: Multitask learning as question answering." [Link to Document]
Why do neural networks generalize? (BA/SP/MA)
It was recently argued in [4] that this happens because minima that lead to good generalization performance have more volume in parameter space and are thus more easily found by optimization procedures. The purpose of this project is to understand what kinds of hypothesis classes lead to such behavior [5, 6]. In this project you will investigate the relationship between volume in parameter space and the shape of decision boundaries of DNNs. Initially, this can be done through simulation experiments, which might then inform a mathematical theory. As a starting point you will study [7] which might be useful, as it uses volumetric arguments to investigate the number of linear regions realized by a DNN.
Type of project: 40%-100% algorithms/programming/simulations, 0%-60% mathematical theory; depending on student's interests
Prerequisites: Programming and visualization skills, mathematical background in geometry
Supervisor: Clemens Hutter
Professor:
Helmut Bölcskei
References:
[1] D. Elbrächter, D. Perekrestenko, P. Grohs, and H. Bölcskei, “Deep neural network approximation theory,” IEEE Transactions on Information Theory, vol. 67, no. 5, pp. 2581–2623, May 2021, doi: 10.1109/TIT.2021.3062161. [Link to Document]
[2] C. Zhang, S. Bengio, M. Hardt, B. Recht, and O. Vinyals, “Understanding deep learning requires rethinking generalization,” in The Fifth International Conference on Learning Representations, Feb. 2017. [Link to Document]
[3] W. R. Huang et al., “Understanding generalization through visualizations,” in I Can’t Believe It’s Not Better! Workshop at the 34th International Conference on Neural Information Processing Systems, 2020. [Link to Document]
[4] P. Chiang et al., “Loss landscapes are all you need: Neural network generalization can be explained without the implicit bias of gradient descent,” in The Eleventh International Conference on Learning Representations, 2023. [Link to Document]
[5] H. Shah, K. Tamuly, A. Raghunathan, P. Jain, and P. Netrapalli, “The pitfalls of simplicity bias in neural networks,” in Proceedings of the 34th International Conference on Neural Information Processing Systems, 2020, pp. 9573–9585. [Link to Document]
[6] A. G. Wilson and P. Izmailov, “Bayesian deep learning and a probabilistic perspective of generalization,” in Proceedings of the 34th International Conference on Neural Information Processing Systems, 2020, pp. 4697–4708. [Link to Document]
[7] B. Hanin and D. Rolnick, “Deep ReLU networks have surprisingly few activation patterns,” in Proceedings of the 33rd International Conference on Neural Information Processing Systems, Oct. 2019, pp. 361–370. [Link to Document]
Approximation rates of transformer networks (SP/MA)
The goal of this project is to derive a mathematical theory for transformer neural networks. Concretely, you would try to understand the fundamental limits of their sequence-to-sequence approximation properties in the spirit of Kolmogorov exponents à la [4], starting from the universal approximation results in [5].
Type of project: 100% theory
Prerequisites: Strong mathematical background
Supervisor: Clemens Hutter
Professor:
Helmut Bölcskei
References:
[1] A. Vaswani et al., “Attention is all you need,” in Proceedings of the 31st International Conference on Neural Information Processing Systems, 2017, pp. 6000–6010. [Link to Document]
[2] J. Devlin, M.-W. Chang, K. Lee, and K. Toutanova, “BERT: Pre-training of deep bidirectional transformers for language understanding,” in Proceedings of the 2019 Conference of the North American Chapter of the Association for Computational Linguistics: Human Language Technologies, Volume 1 (Long and Short Papers), Jun. 2019, pp. 4171–4186. [Link to Document]
[3] T. B. Brown et al., “Language models are few-shot learners,” in Proceedings of the 34th International Conference on Neural Information Processing Systems, Jul. 2020, pp. 1877–1901. [Link to Document]
[4] D. Elbrächter, D. Perekrestenko, P. Grohs, and H. Bölcskei, “Deep neural network approximation theory,” IEEE Transactions on Information Theory, vol. 67, no. 5, pp. 2581–2623, May 2021, doi: 10.1109/TIT.2021.3062161. [Link to Document]
[5] C. Yun, S. Bhojanapalli, A. S. Rawat, S. J. Reddi, and S. Kumar, “Are transformers universal approximators of sequence-to-sequence functions?,” in The Eighth International Conference on Learning Representations, Feb. 2020. [Link to Document]
Acoustic sensing and trajectory estimation of objects flying at supersonic speed (with industry) (SP)
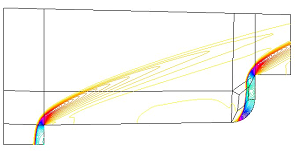
The goal of this project is to investigate new techniques for the task at hand, such as linearization of non-linear systems of equations, least squares fitting, and neural network driven machine learning. Existing hardware and algorithms provide a starting point for the project, which will be carried out in collaboration with an industry partner called SIUS (located in Effretikon, Zurich). SIUS offers close supervision and the possibility to use hardware and a test laboratory.
About the industry partner: SIUS is the world’s leading manufacturer of electronic scoring systems in shooting sports. The company is specialized in producing high speed and high precision measurement equipment capable of measuring projectile position and trajectory and has been equipping the most important international competitions including the Olympic Games for decades.
Type of project: 20% literature research, 20% theory, 50% implementation/programming, 10% experiments
Prerequisites: Solid mathematical background, knowledge of SciPy, Matlab or a similar toolset, ideally knowledge on (deep) neural networks
Supervisor: Michael Lerjen, Steven Müllener
Professor:
Helmut Bölcskei
References:
[1] SIUS Homepage [Link to Document]
Separability of structured data (SP)
The goal of this project is to extend Cover's framework [1] to structured data, such as, e.g., data points that are grouped in tuples [2], as illustrated in the figure, or that exhibit sparsity in the sense of compressed sensing theory [3, 4].
Type of project: 100% theory
Prerequisites: Strong mathematical background
Supervisor: Konstantin Häberle
Professor:
Helmut Bölcskei
References:
[1]
T. Cover, “Geometrical and statistical properties of systems of linear inequalities with applications in pattern recognition,” IEEE Transactions on Electronic Computers, vol. 14, no. 3, pp. 326-334, 1965.
[Link to Document]
[2] P. Rotondo, M. Lagomarsino, and M. Gherardi, “Counting the learnable functions of geometrically structured data,” Physical Review Research, vol. 2, no. 2, p. 023169, 2020. [Link to Document]
[3] E. Candès, J. Romberg, and T. Tao, “Robust uncertainty principles: Exact signal reconstruction from highly incomplete frequency information,” IEEE Transactions on Information Theory, vol. 52, no. 2, pp. 489–509, 2006. [Link to Document]
[4] D. Donoho, “Compressed sensing,” IEEE Transactions on Information Theory, vol. 52, no. 4, pp. 1289–1306, 2006. [Link to Document]
Complexity of ꞵ-expansions (MA/SP)
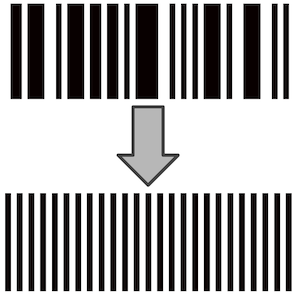
A fundamental question remains: Which representation is more advantageous in terms of storage on a computer, the binary expansion, the decimal expansion or β-expansions? In prior work by our group, it was shown that β-expansions can theoretically be compressed down to the same limit as the binary expansion. We now want to investigate how compression of β-expansions works in practice, e.g. when using classical compression algorithms such as Huffman coding, Lempel-Ziv-Welch, LZ77, etc. In this project, you would first study theoretical results on the compressibility of β-expansions, and then investigate the practical performance of compression algorithms on β-expansions.
Type of project: 30% theory, 70% simulation
Prerequisites: Good programming skills, knowledge in machine learning and appetite for functional analysis
Supervisor: Valentin Abadie
Professor:
Helmut Bölcskei
References:
[1]
G. Kallós, "On some problems of expansions investigated by P. Erdős et al.," Annales Univ. Sci. Budapest, Sect. Comp., Vol. 45, pp. 239-259, 2016.
[Link to Document]
[2] I. Daubechies, R. A. DeVore, C. S. Güntürk, and V. A. Vaishampayan, "A/D conversion with imperfect quantizers," IEEE Transactions on Information Theory, vol. 52, no. 3, pp. 874-885, March 2006, doi: 10.1109/TIT.2005.864430. [Link to Document]
Learning cellular automaton transition rules with transformers (MA)
A cellular automaton (CA) is a discrete dynamical system consisting of a regular lattice in one or more dimensions with cell values taken from a finite set. The cells change their states at synchronous discrete time steps based on a transition rule [1]. Despite the simplicity of the CA model, it can exhibit complex global behavior. With suitably chosen transition rules, cellular automata can simulate a plethora of dynamical behaviors [2, 3]. The inverse problem of deducing the transition rule from a given global behavior is extremely difficult [4]. In this project, you will investigate the possibility of training transformers [5] to learn CA transition rules.
Type of project: 30% theory, 70% implementation
Prerequisites: Good programming skills, knowledge in machine learning
Supervisor: Yani Zhang
Professor:
Helmut Bölcskei
References:
[1]
J. Kari, “Theory of cellular automata: A survey,” Theoretical computer science, 334(1-3):3–33, 2005.
[Link to Document]
[2] T. Toffoli and N. Margolus, “Cellular automata machines: A new environment for modeling,” MIT press, 1987. [Link to Document]
[3] A. Adamatzky, “Game of life cellular automata,” vol. 1, Springer, 2010. [Link to Document]
[4] N. Ganguly, B. K. Sikdar, A. Deutsch, G. Canright, and P. P. Chaudhuri, “A survey on cellular automata,” 2003. [Link to Document]
[5] A. Vaswani, et al., “Attention is all you need,” Advances in Neural Information Processing Systems 30, 2017. [Link to Document]
Continuous-time recurrent neural networks in Banach spaces (SP)
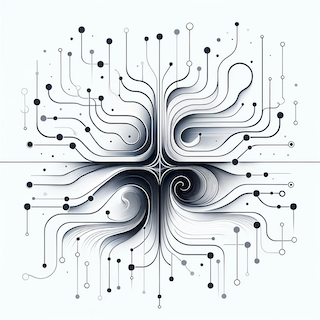
Although there exists a rich literature on approximation-theoretic properties of CTRNNs, there is a lack of explicit constructive results. The standard statements in the literature often implicitly rely on the fundamental theorem of approximation for neural networks [5, 6]. The goal of the present project is to fill this gap by explicitly constructing CTRNNs that approximate functions in Banach spaces, including Besov spaces, Sobolev spaces, spaces of analytic functions, Hölder spaces, and Lipschitz spaces. You will approach this problem by studying how bases in such spaces, such as bases of sinusoids, wavelets, or splines, can be approximated by CTRNNs.
Type of project: 100% theory
Prerequisites: Strong mathematical background
Supervisor: Thomas Allard
Professor:
Helmut Bölcskei
References:
[1]
J. Hopfield, “Neural networks and physical systems with emergent collective computational abilities,” Proc. Nat. Acad. Sci. United States Amer., vol. 79, no. 8, pp. 2554–2558, Apr. 1982.
[Link to Document]
[2] P. Dayan and L. F. Abbott, "Theoretical neuroscience: Computational and mathematical modeling of neural systems," MIT press, 2001. [Link to Document]
[3] M. Kennedy and L. Chua, “Neural networks for nonlinear programming,” IEEE Trans. Circuits Syst., vol. 35, no. 5, pp. 554–562, May 1988. [Link to Document]
[4] E. D. Sontag, “A learning result for continuous-time recurrent neural networks,” Systems and Control Letters, vol. 34, no. 3, pp. 151–158, June 1998. [Link to Document]
[5] K.-I. Funahashi and Y. Nakamura, “Approximation of dynamical systems by continuous time recurrent neural networks,” Neural Networks, vol. 6, pp. 801–806, Jan. 1993. [Link to Document]
[6] G. Cybenko, “Approximation by superpositions of a sigmoidal function,” Mathematics of Control, Signals and Systems, vol. 2, no 4, pp. 303-314, 1989. [Link to Document]
Finite-precision neural networks (MA)
The first step of the project is to generalize the theory developed in [1, 2] to neural networks with both weights and signals in all layers of finite precision and to establish fundamental limits on function approximation through such networks. Specifically, the new theory should be able to answer the question of how a given overall bit budget for operating the neural network should be distributed across the weights and signals in the network so as to minimize the end-to-end approximation error for a given task. The second major goal of the project is to identify function classes for which approximation through finite-precision neural networks achieves the fundamental limits identified in the first part.
The project is carried out in collaboration with Dr. Van Minh Nguyen in the form of an internship at Huawei Labs in Paris.
Type of project: 70% theory, 30% simulation
Prerequisites: Strong mathematical background and good programming skills
Supervisor: Weigutian Ou
Professor:
Helmut Bölcskei
References:
[1]
H. Bölcskei, P. Grohs, G. Kutyniok, and P. Petersen, "Optimal approximation with sparsely connected deep neural networks," SIAM Journal on Mathematics of Data Science, vol. 1, no. 1, pp. 8–45, 2019.
[Link to Document]
[2] D. Elbrächter, D. Perekrestenko, P. Grohs, and H. Bölcskei, "Deep neural network approximation theory," IEEE Transactions on Information Theory, vol. 67, no. 5, pp. 2581–2623, May 2021. [Link to Document]
General signal denoising (MA/SP)
This project seeks to explore the signal denoising problem under more general assumptions on the data structures, while imposing low dimensionality in a suitable sense. The first goal of the project is to identify the best possible performance achievable by any denoising algorithm, and the second is to design algorithms that can provably attain this best performance.
Type of project: 80% theory, 20% simulation
Prerequisites: Strong mathematical background
Supervisor: Weigutian Ou
Professor:
Helmut Bölcskei
References:
[1]
P. J. Bickel,
"Minimax estimation of the mean of a normal distribution when the parameter space is restricted," The Annals of Statistics, 9(6): 1301–1309, 1981.
[Link to Document]
[2] D. L. Donoho, R. C. Liu, and B. MacGibbon, "Minimax risk over hyperrectangles, and implications," The Annals of Statistics, 18(3): 1416–1437, 1990. [Link to Document]
[3] D. L. Donoho and I. M. Johnstone, "Minimax risk over 𝓁p-balls for 𝓁q-error," Probability Theory and Related Fields, 99(2):277–303, 1994. [Link to Document]
[4] D. L. Donoho and M. Gavish, "Minimax risk of matrix denoising by singular value thresholding," The Annals of Statistics, 42(6): 2413–2440, 2014. [Link to Document]
The "logic" behind transformers (MA)
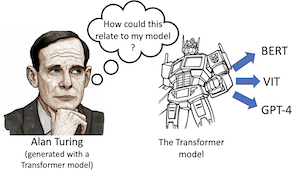
In this project, you will familiarize yourself with literature on links between Turing machines and neural networks. You would then establish direct connections between the transformer architecture and Turing machines, with the goal of understanding better how transformers simulate Turing machines.
Type of project: 100% theory
Prerequisites: Knowledge in neural network theory and theoretical computer science, appetite for theory in general
Supervisor: Valentin Abadie
Professor:
Helmut Bölcskei
References:
[1]
A. Vaswani, N. Shazeer, N. Parmar, J. Uszkoreit, L. Jones, A. N. Gomez, L. Kaiser, and I. Polosukhin, "Attention is all you need," Advances in Neural Information Processing Systems, 2017.
[Link to Document]
[2] S. Bhattamishra, A. Patel, and N. Goyal, "On the computational power of transformers and its implications in sequence modeling," arXiv preprint arXiv:2006.09286, 2020. [Link to Document]
[3] H. T. Siegelmann and E. D. Sontag, "On the computational power of neural nets," Journal of Computer and System Sciences, vol. 50(1), pp. 132–150, 1995. [Link to Document]
Reconstruction and classification of eclectrocardiograms with transformers (BA/SP/MA)
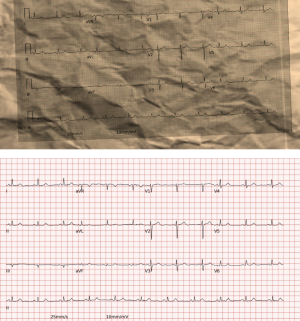
In this project, you will combine these two architectures to reconstruct and classify multivariate time series in an end-to-end manner. Then, the combined architecture will be evaluated on the electrocardiogram (ECG) dataset provided by the George B. Moody PhysioNet Challenge 2024 [5]. Specifically, you will reconstruct and classify ECG signals from low-quality paper ECGs collected in the Global South.
Type of project: 30% theory, 70% programming
Prerequisites: Programming, machine learning, background in signal processing is a plus
Supervisor: Clemens Hutter, Yani Zhang
Professor:
Helmut Bölcskei
References:
[1]
A. Vaswani et al., “Attention is all you need,” Advances in Neural Information Processing Systems, 30, 2017.
[Link to Document]
[2] I. Goodfellow, Y. Bengio, and A. Courville, “Deep Learning,” MIT press, 2016. [Link to Document]
[3] S. Mallat, “Group invariant scattering,” Communications on Pure and Applied Mathematics, vol. 65, no. 10, pp. 1331-1398, 2012. [Link to Document]
[4] T. Wiatowski and H. Bölcskei, “A mathematical theory of deep convolutional neural networks for feature extraction,” IEEE Transactions on Information Theory, vol. 64, no. 3, pp. 1845-1866, 2018. [Link to Document]
[5] Digitization and Classification of ECG Images: The George B. Moody PhysioNet Challenge, 2024 [Link to Document]